Problems
Big Data
Never before in history data is generated and collected at such high volumes as it is today. For the analysis of large data sets to be effective, it is important to include the human in the data exploration process and combine the flexibility, creativity, and general knowledge of the human with the enormous storage capacity and the computational power of today's computers. Visual Analytics helps to deal with the flood of information by integrating the human in the data analysis process, applying its perceptual abilities to the large data sets. Presenting data in an interactive, graphical form often fosters new insights, encouraging the formation and validation of new hypotheses for better problem-solving and gaining deeper domain knowledge. Visual analytics techniques have proven to be of high value in exploratory data analysis. They are especially powerful for the first steps of the data exploration process, namely understanding the data and generating hypotheses about the data, but they also significantly contribute to the actual knowledge discovery by guiding the search using visual feedback.
Lack of Methods to Auto Detect Patterns and Anomalies
For examples:
-
Without learning from models on automatically detect exceptions in a multi-dimensional IoT (Internet of Things) time series.
-
Traditional anomaly detection technologies analyze exceptions, but often neglect the anomalies that occur consecutively without any time gaps.
-
To identify security attack patterns in high-dimensional network data, analysts often use a scatter plots (port graphs) for attack detection. If the ports are scanned sequentially, the attacks become visible as diagonal lines. If the ports are accessed in randomized order, the port scans are not visible in the graphs.
Important information often lost from large volume of text data (email, review, tweets)
Today’s sentiment analysis technologies analyze customer reviews, but typically do not allow real-time detection of critical issues (topics: such as computer, printer) in the streams of customer opinions. Customer satisfaction can only be maximized if the stream of customer reviews is analyzed in real time to identify periods of increased negative feedback, to analyze their root-causes, and to take immediate action.
​
​
​
​
​
​
Our Solutions:
Accessing your data from datasets (SQL) and using our statistics algorithms, machine learning models to arrive an answer. The result will be presented in a visual analytics dashboard. Customers can iteratively see the insight of their data and identify patterns and anomalies.
Our Platform:
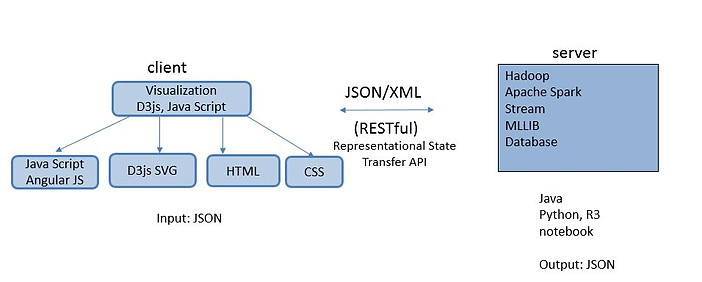
Some Interesting Samples:
• Auto-Detect Anomalies in Market Campaign Forecasting
Created “Consecutive Anomaly Auto-Detection” dashboard from large campaign forecast data stream using real-time learning models. Customers are able to instant catch a series of performance anomalies
​
• Optimize energy consumption (Internet of Things)
Invented “Exception Bands” to optimize and predict energy consumption (temperature, lighting, etc.)
Created learning models on a large IoT time series. Energy savings improved 18%. (HP Smart Office).
​
• Perform security threat detection
Created “automated Threat Detection” ML models on a Hadoop/Spark platform. Built distance matrices to search known threats having similar
behaviors (Port Scan). Using classification, hierarchical clustering to find unknown threats (C&C servers and Botnets).
Adopted by HP “Cyber Defense Center”. Discovered millions malicious IPs from 300+ network features.
• Balance SaaS customer workflow without exceeding capacity limit
Employ "Peak Preserving" to protect SaaS customers (Adidas, Airbus, Goodyear) not to overuse resources.
​
• Combine "Words" into "Topics" to solve critical customer concerns. (Natural Language Processing)
Emails and Tweets from surveys.
• Conduct user-centered, interactive visualization (Dashboard)
Use for BI Operation (i.e., Southwestern, Halliburton and supply chains)